Radar uptake in vehicles will continue its increase, driven both by an increase in the number of adopting cars and by the radar content per vehicle. Both trends are driven by the increasing adoption of ADAS and will be sustained and strengthened in the longer term by the emergence of highly automated or autonomous driving.
For level 2 and above cars and trucks, an IDTechEx market report forecasts that automotive radar unit sales (SRR, MRR, and LRR together) will increase from 55M units in 2019 to 223M and 400M in 2030 and 2040, respectively. This is a whopping increase of 4 and 7 times.
The research body justifies its long-term forecasts, because its “models suggest that highly automated and autonomous cars will take time to develop.”
It explains: “Indeed, we forecast that level 4 and level 5 passenger cars will reach 5M and 0.5M units in 2030 and 24M and 16M in 2040, respectively.
“It is a good time to be in the radar business not just because the market will grow, but also because the innovation at every level is taking place at a fast pace. In the remainder of this article, we outline some key innovation trends.”
A recent IDTechEx’s report, titled: “Automotive Radar 2020-2040: Devices, Materials, Processing, AI, Markets, and Players” mirrors a comprehensive technology roadmap, examining the technology at the levels of materials, semiconductor technologies, packaging techniques, antenna array, and signal processing/AI.
Examining the latest product innovation, the reports identifies and reviews promising start-ups worldwide, building a short- and long-term forecast model covering the period between 2019 to 2040. Also the market- in unit numbers and value- is segmented by the level of autonomy and by passenger vehicles, shared vehicles, and trucks.
Another report from IDTechEx , “Autonomous Cars and Robotaxis 2020-2040: Players, Technologies and Market Forecast”, offers a full analysis of private and shared autonomous cars and robotaxis. It has a detailed realistic model, predicting the adoption of private and shared highly automated and autonomous car, offering market value projections segmented by hardware and software component.

Hardware Trends and Innovations
From GaAs to SiGe to Si: Let’s start with the semiconductor. We have already seen a complete transition for die-on-board wire-bonded GaAs to wafer-level-packaged SiGe. This transition started in 2008/9 and is largely completed. The technology node is 180nm with some working on 130nm too. We are now at the start of another transition, however. This time from SiGe towards Si CMOS or SOI.
Si CMOS and smaller tech nodes: As the demand volume grows, it becomes economically justified to allocate more advanced technology nodes to radar silicon IC production. These smaller nodes, in turn, enable silicon-based ICs to compensate for silicon’s inherent lower mobility. The main nodes of choices today are 40/45nm, 28nm, and 22nm. Some are even going for 16nm. This trend towards ever smaller nodes will continue with growing volume demand.
From individual dies to fully integrated all-in-one ICs: The transition to CMOS will bring with it further function integration possibilities. In the pre-2010 era, GaAs die were assembled as chip-on-board (COB). Post-2012, we witnessed SiGe WLP packages, but each Rx and Tx unit had a separate package. From 2014 to 2017, we saw the integration of multiple Rx/Tx in a single package together with some monitoring functions. Next, the waveform generation was also integrated. Now- with the rise of Si CMOS or SOI radar ICs- we are seeing the integration of the microcontroller and the DSP within a single package. This is results in a fully-integrated all-in-one radar IC – i.e., power management IC is still off-IC.

Advanced packages and AiP: the first generation of automotive radar, the radar board was separated from the processing and power management board. In the second generation, they were still separated but the RF board had shrunk in size. In the current generation, it is common to have a hybrid digital/RF board.
An emerging trend to integrate the antenna in the package (AiP). This trend in radar development is seen for both automotive and non-automotive applications. Indeed, OSATs have already qualified automotive-grade MRR 77GHz eWLB AiP packages. In this case, the antenna is integrated in the Cu layer of the RDL (re-distribution layer).
In another case, AiP for 122GHz silicon-based radars with six antennas integrated in a package are also launched, creating a single chip solution which targets patient or elderly monitoring, in-cabin monitoring, and similar applications. Note that the antenna sizes and spacing will shrink with frequency, making AiP physically possible even for larger antenna arrays.
Note that the trends towards AiP are also seen on mmWave 5G package. The two developments will have much in common and will be synergic.
In general, the frequency of automotive radar has shifted up towards the 77-81GHz range. As we go higher in frequency, the radial speed resolution and the angular separation capability improve. Implementing a wider bandwidth will become easier as the wide bandwidth will be a smaller portion of the centre frequency. The wider bandwidth will translate directly into high range resolution.
Minimising transmission loss: However, higher frequency also means higher transmission losses, both at the board and within the package levels. To mitigate this, three strategies are pursued: (1) deploy low-loss RF materials such as ceramic-filled PTFEs (other candidates include LCP, LTTCC, glass, etc), (2) deploy ultra-smooth conductive traces, and (3) shorten signal travel distances by integrating more within the package.
The latter approach entails packaging challenges. The material set will need to be improved to minimize transmission loss. This includes the EMC but also the dielectric layers within the RDL or high-density substrates. The choice of the packaging technique is also crucial with fan-out typically showing superior results than BGA and flip-chip.

Towards large antenna arrays: the Tx/Rx count is increasing. The Gen-1 SRR radars had 1Tx/2Rx. By Gen 4 and 5 premium radars this had reached 4Tx/6Rx and 12Tx/16Rx, previously. This trend will continue. Indeed, start-ups are pushing the performance envelope, developing radar systems with 48Tx/48Tx and even 72Rx/72Tx. The architecture that supports large antenna arrays plays a vital role and will likely consist of beamforming ICs in conjunction with radar transceivers.
The trend towards large antenna arrays is critically important because it drives down the azimuth and elevation resolution to below 1 and 2deg, respectively. This is a crucial development because it allows the radar to see more precisely and to separate out objects in more detail. It also densifies the point cloud, allowing much more advanced AI-based signal processing to do multi-object recognition or tracking (more on this later).
The weather and light condition independence, and the ability to detect velocity information per frame make radar superior to time-of-flight lidars (most mature lidar tech of today). Lidars however remain superior in terms of, for example, resolution. Will the large antenna arrays together with the accompanied higher resolution and densified point cloud blur this differentiation of lidars? Perhaps not easily since, as shown below, lidar remains superior despite these recent advances. On the other hand, can the long-term development of SWIR (1550nm) FMCW lidar pose a long-term threat to radars? To learn more about lidars please see the IDTechEx report, “Lidar 2020-2030: Technologies, Players, Markets & Forecasts”. This is the most comprehensive and authoritative report on the topic.
The trend toward large antenna areas together with novel architectures involving beamforming ICs mirrors developments in mmWave 5G, whose main ideas are based on antenna gain and spatial beamforming. In automotive radar, these principles will enable electronically scanned radar systems.
There are of course more trends. To learn the latest, to gain a deeper insight, to find out about the players and innovations, and to obtain our granular detailed market forecasts please see the IDTechEx report, “Lidar 2020-2030: Technologies, Players, Markets & Forecasts”.
Software Trends and Innovations
Hardware developments are important, but they are only a part of the story. In a typical radar, the incoming signal is first digitized, and three rounds of Fast Fourier Transform are performed to create the range and velocity data matrix and to find the presence of objects through signal peak detection.
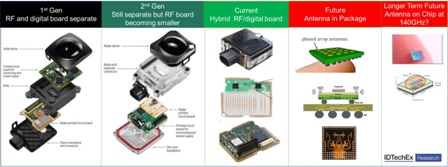
To get the angle, one typically uses multiple antennas, converting the phase difference which stems from the physical separation of the antennas into an angle of arrival estimate. Given the multi-array arrangement, the output data is now typically a 3D data cube. Here, roughly speaking, the size of the antenna array determines the resolution.
One can easily imagine that the data rates rapidly scale up with increasing antenna array size – i.e., 240-360Gbit/s with 16-24Rx/8-12Tx radar. This will result in challenges in data management which we will not discuss further here.
Towards 3D multi-object detection and tracking: In autonomous driving, we need to do much more: one needs to detect and track multiple objects, preferably in 3D. To this end, one requires some deep learning or CNN based train algorithm.
Radar has many limitations. For example, the sparsity of the point cloud of traditional radar is sparse. To appreciate this limitation, consider that video and lidar generate 2M and 165k voxels per scan, whereas radar generates 10k. In other words, radar is like a 10kpixel camera in comparison with a standard 2MPixel camera. To remedy this challenge, massive antenna arrays will be required (see the hardware trend section).
Nonetheless, the use of radar to do object detection and tracking is a growing frontier of research. Radar datasets for autonomous driving are being developed and made available, sometimes as open-source – e.g., Astyx. Semi-manual labelling mechanisms are being developed, to accelerate and reduce the cost of training data annotation.

In one approach, camera-based machine vision is first used to detect objects, drawing a bounding box around it. Next, lidar data is used to give depth to the bounding box, separating items within the bounding box by depth. Finally, the radar clusters falling within the bounding box of the camera+lidar detect objects are automatically annotated. This way, the time to tag a cluster is reduced to 0.02s (vs 10min for manual). Of course, this will require powerful GPUs. More importantly, though is that accuracy is not yet as high (72% vs 99% for manual).
Of particular note are recent results by Delft University showing target-wise average F1 scores of 0.7. To achieve these leading results, the researchers deployed both low-level and target-level data to train the algorithm. The main insight here was that each object class has a unique set of movement signatures which are buried in the 3D cube.
Despite this and similar progress, the ability of radar alone remains limited. To overcome, radar will be used as part of a sensor fusion set up involving lidar and cameras. This will limit to many questions and challenges – e.g., early vs late fusion. In the longer term, data fusion will be prevalent. We may write more on this topic in subsequent articles.
Aside demonstrating that radar technology has entered a golden age of innovation and growth, one development axis is to create ubiquitous low-cost AiP single-chip radars. Another is to scale the antenna array and system architecture to improve resolution and density point cloud.
In parallel, the radar is moving from detecting velocity, angle, and location to detecting and tracking 3D objects. In the automotive sector, the market is fast growing supported by strong multiplying trends. The radar is also setting the foundation to become a commonplace sensing technology in many day-to-day activities including human monitoring, gesture recognition, etc.